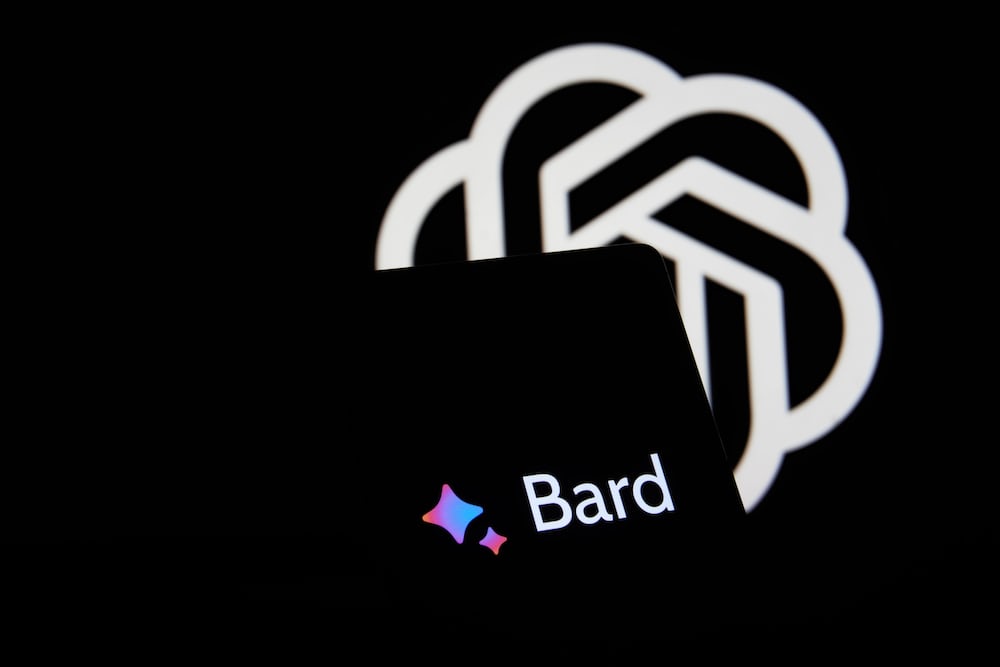
In an era defined by technological advancements, the integration of Generative Artificial Intelligence (Generative AI) has become increasingly prevalent, reshaping various sectors, including government services.
Generative Artificial Intelligence (Generative AI) (abbreviated as “AIGC” for AI Generative Content) refers to a production method that uses artificial intelligence to automatically generate new, contextually relevant content.
AIGC tools utilize deep learning methods to learn existing knowledge, and when prompted, generative artificial intelligence can create new content based on statistical models.
The objective of this article is to assess and understand the strengths and weaknesses of both government chatbots and AIGC technology. The analysis likely involves evaluating their performance, capabilities, and areas of improvement to gain insights into the effectiveness of these systems.
Introduction
In November 2022, the American company OpenAI launched an artificial intelligence dialogue chatbot, ChatGPT. The launch of ChatGPT sparked a wave of interest in Large Language Models (LLMs).
Similarly, AIGC has also been rapidly adopted in various fields. This integration aims to facilitate rapid and personalized responses, significantly reducing the workload of public service personnel.
In the United States, Arizona’s Department of Economic Security has deployed a chatbot named “Dave” to assist citizens in understanding public finance information. Likewise, the Maryland Department of Labor utilizes “Dayne”, a chatbot providing information related to unemployment benefits. Finland has introduced “Kamu”, a chatbot aimed at offering citizen services, demonstrating the broad applicability of these technologies in various administrative functions.
Also, in the United Kingdom, the National Health Service (NHS) has integrated AIGC into its chatbot, “Ada Health”, to offer health-related advice, including vaccine information and diagnosis, thus facilitating personalized health assessments. Moreover, Estonia has launched a virtual assistant called “Suve”, designed to provide accurate and reliable information in response to public inquiries.
In this article, we will be investigating the comparative analysis of the ability of two large language model (LLM)-based chatbots – ChatGPT and Wenxin Ernie by posing various questions of different aspects and complexities. By utilizing text analysis, index evaluation, joint experimentation, and Natural Language Processing (NLP) technologies, the study delves deeply into exploring these two LLMs and gathering data.
Purpose Of The Experiment
Generative AI models are a subset of large language models (LLMs). For example, generative pre-trained transformer (GPT). GPT-3 is trained on 175 billion parameters, while GPT-4 is trained on one trillion parameters. An intermediary version, GPT-3.5, is specifically trained to predict the next word in a sequence using a large dataset of Internet text. It is the model that underpins the current version of ChatGPT.
However, distinct from traditional AI systems, which are typically rule-based or rely on predefined datasets, generative AI models possess the unique ability to create new content that is original and not explicitly programmed. This can result in outputs that are similar in style, tone, or structure to the prompt instruction.
Therefore, if designed thoughtfully and developed responsibly, generative AI has the potential to produce smarter policymaking, reimagined service delivery, and more efficient operations. AI and data analytics can enhance the effectiveness of policymaking, providing decision-makers with tools to deliver more value.
The main purpose of this experiment is to explore whether the existing AIGC technology can be effectively applied to robot government affairs chat and propose feasible optimization directions. This study involves a preliminary textual analysis of the responses generated by the two models, including similarity analysis, word frequency analysis, responsibility analysis, communicative analysis, and user-friendliness analysis.
Methodology
Test questions were designed based on procedural problems and complex problems, and the keywords of each service section (such as Retirement care, Public security, Education, etc.) and continuous follow-up questions were conducted.
Initially, these test questions were inputted into the Guangdong Government Affairs Chatbot, as well as the two LLM models being considered to obtain corresponding results and conduct text analysis.
The preliminary text analysis is conducted based on the text data generated from AIGC answers, including topic analysis, similarity analysis, responsibility analysis, and sentiment analysis.
However, during this testing, the response of the Guangdong Government Affairs Chatbot often provides links directly, resulting in vague and general answers, making it difficult to provide satisfactory responses to public government affairs questions.
Through the aforementioned text analysis techniques, it was easier to delve into text data, uncover the structure and content of artificial intelligence documents, and identify the core issues and specific concerns in policy texts.
This allows for a better understanding of how artificial intelligence is discussed and applied in the field of governance. These analyses not only reveal high-frequency keywords and topics but also uncover the deeper meanings and public sentiments in the text, providing data support for policy-making and public services.
Research Results and Conclusion
Recently, the intelligence and informatization of government services have garnered increasing attention from the public. How to use advanced technology to enhance the quality and efficiency of government services has become a crucial topic in government innovation.
ChatGPT and Ernie, as prominent examples of Generative AI, are attracting more attention for their potential applications in government Q&A. This section aims to verify the accuracy and usefulness of ChatGPT and Ernie in handling government-related questions, providing decision support and references for government departments to integrate AI into digital services.
The recent popularity of interactive models such as ChatGPT and Ernie has broken people’s stereotypical impressions of machine conversations, with AIGC becoming a crucial tool for white-collar professionals. Therefore, how to apply AIGC to enhance the quality and efficiency of government services has become a pivotal topic in governmental innovation.
This research, based on text analysis, conducted a comprehensive analysis of the response texts from ChatGPT and Ernie in four dimensions namely:
- topic analysis,
- similarity analysis,
- conscientiousness analysis, and
- sentiment analysis.
In addressing responses to complex questions, both LLM models exhibited specific response styles, including the use of encouraging language and expressions of positive emotions. Using ChatGPT as a reference, Ernie’s responses demonstrated higher conscientiousness and a more balanced expression of rational emotions.
Therefore, by introducing three major scoring dimensions – conscientiousness, communicativeness, and populism – and comparing the overall performance of the two AIGC models, the study elucidates public preferences in governmental conversations, indicating optimization directions for government robots.
Additionally, it should focus on responding to the emotional needs of inquirers, and use encouraging language to mitigate negative emotions.
Source 1
https://arxiv.org/pdf/2312.02181
Source 2
https://www.ncbi.nlm.nih.gov/pmc/articles/PMC10606429/
One thought on “How To Use Generative AI (Large Language Models) in Government Chatbots”